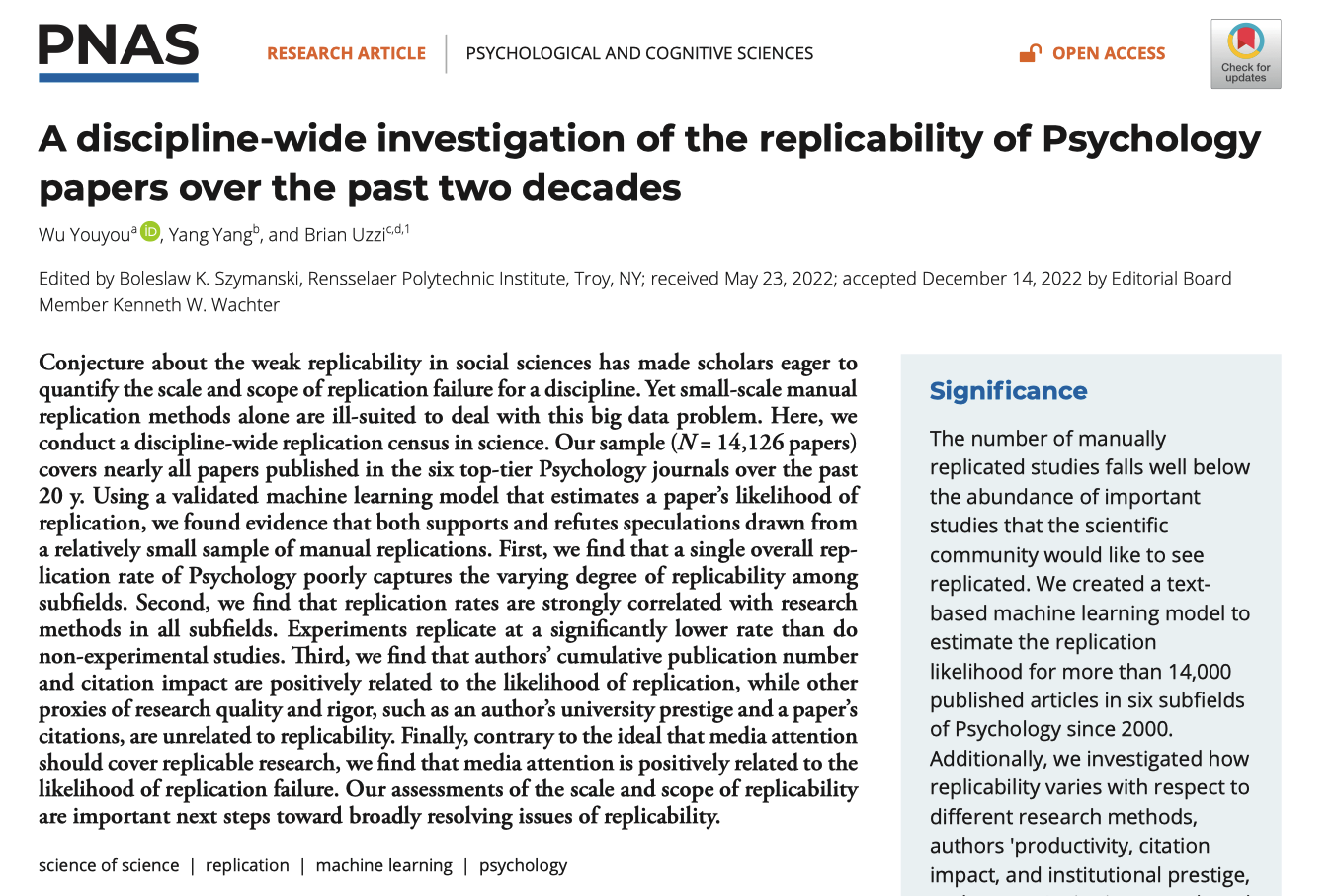
PNAS | 14000+篇心理学顶刊论文可复现性调研
”可复现研究的数量远远低于科学界期望,我们创建了一个基于文本数据的机器学习模型,估计了自2000年以来心理学六个子领域中发布的超过14,000篇文章的可复现性分析。此外,我们还调查了可复现性与不同的研究方法、作者的生产力、引用影响力和机构声誉、论文的引用增长和社交媒体覆盖率有关的变化。我们的研究结果有助于建立大规模的经验模式,以便为推进复现研究提供依据。The number of manually replicated studies falls well below the abundance of important studies that the scientific community would like to see replicated. We created a text-based machine learning model to estimate the replication likelihood for more than 14,000 published articles in six subfields of Psychology since 2000. Additionally, we investigated how replicability varies with respect to different research methods, authors 'productivity, citation impact, and institutional prestige, and a paper’s citation growth and social media coverage. Our findings help establish large-scale empirical patterns on which to prioritize manual replications and advance replication research.“...