代码获取
Kaggle是个很棒的地方,对于数据科学家和机器学习工程师来说,这是一个知识的金矿。可以在同一地点找到由本领域专家带来的高质量,高效,可重现,很棒的代码。自推出以来,它已经举办了164场比赛。这些比赛吸引了来自世界各地的专家和专家加入该平台。结果,每场比赛以及Kaggle提供的大量开源数据集都有许多高质量的笔记本和脚本。
在数据科学之旅的开始,我将去Kaggle查找数据集以练习我的技能。每当我查看其他内核时,我都会对代码的复杂性感到不知所措,然后马上回避。
但是现在,我发现自己花费了大量时间阅读其他笔记本并提交竞赛文件。有时候,有些东西值得您度过整个周末。有时,我会发现简单但致命的有效代码技巧和最佳实践,这些技巧和最佳实践只能通过观察其他专家来学习。
在整个系列中,您会发现我在典型的数据科学工作流程中可能有用的任何内容,包括与通用库相关的代码快捷方式,Kaggle的顶级行业专家遵循的最佳实践等,这些都是我在学习过程中学到的。
1. 只绘制相关系数矩阵的下三角部分
好的相关矩阵可以说明数据集中目标变量之间的相关性
import pandas as pd
df = pd.read_csv('data/melbourne_housing_raw.csv')
df.head()
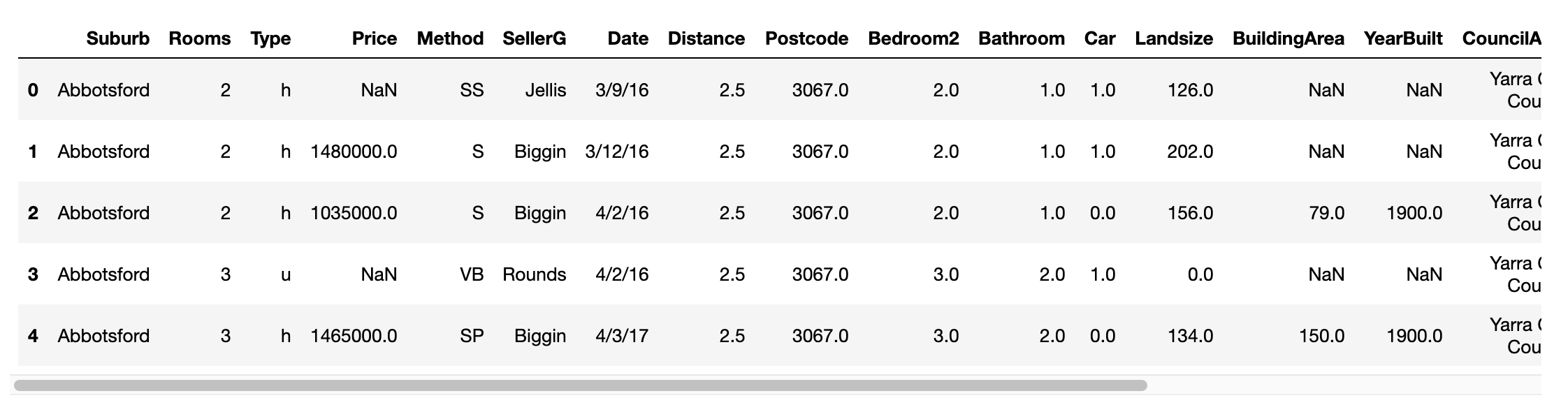
import seaborn as sns
import matplotlib.pyplot as plt
plt.figure(figsize=(16, 12))
cmap = sns.diverging_palette(250, 15, s=75, l=40,
n=9, center="light", as_cmap=True)
sns.heatmap(df.corr(), center=0, annot=True,
fmt='.2f', square=True, cmap=cmap)
plt.show()
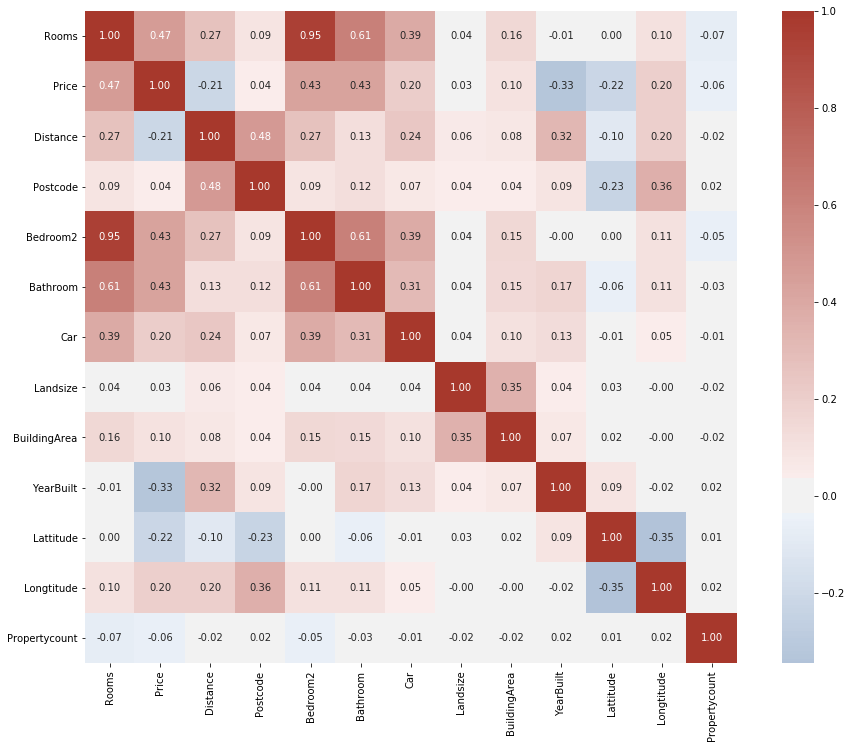
但上图中,数据集中存在大量的特征,导致相似矩阵过于庞大,让人看起来不知所措。
相关矩阵大部分沿主对角线对称,因此它们包含重复数据。 同样,对角线本身也没有用。 让我们看看如何只绘制有用的一半:
import numpy as np
# 计算相关系数
matrix = df.corr()
# 创建遮罩(为了只显示下三角)
mask = np.triu(np.ones_like(matrix, dtype=bool))
# 定制调色板
cmap = sns.diverging_palette(250, 15, s=75, l=40,
n=9, center="light", as_cmap=True)
# 设定图片尺寸
plt.figure(figsize=(16, 12))
# 绘制相似矩阵热力图
sns.heatmap(matrix, mask=mask, center=0, annot=True,
fmt='.2f', square=True, cmap=cmap)
plt.show()
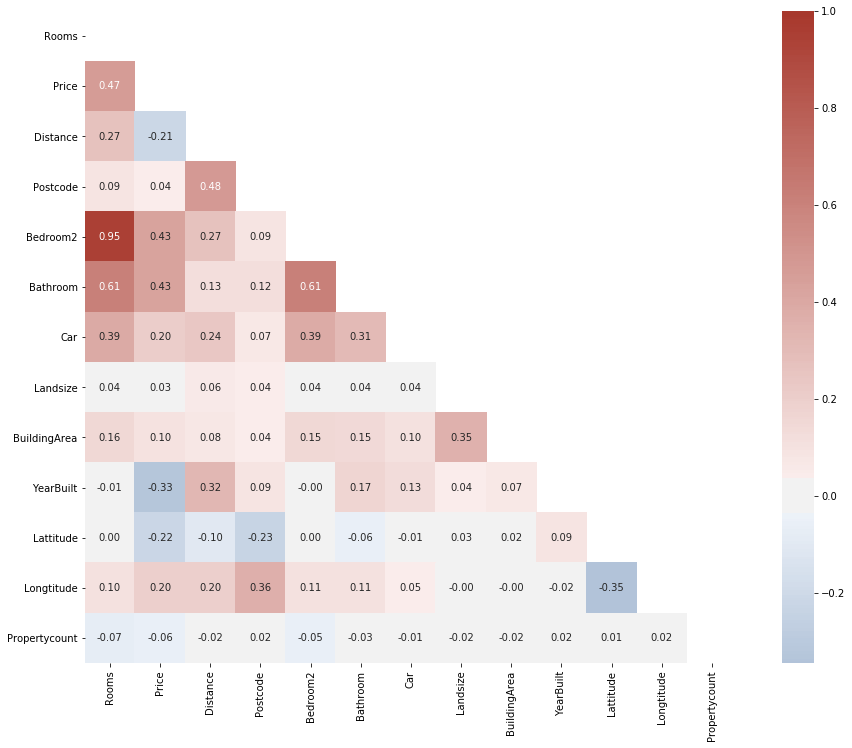
由此产生的可视化图更容易解释并且没有视觉干扰干扰。
- 首先,我们使用DataFrame的.corr方法构建相关矩阵。
- 然后,我们使用dtype设置为bool的np.ones_like函数来创建一个True矩阵,其形状与DataFrame相同:
np.ones((5, 5))
array([[1., 1., 1., 1., 1.],
[1., 1., 1., 1., 1.],
[1., 1., 1., 1., 1.],
[1., 1., 1., 1., 1.],
[1., 1., 1., 1., 1.]])
np.ones_like(np.ones((4, 4)), dtype=bool)
array([[ True, True, True, True],
[ True, True, True, True],
[ True, True, True, True],
[ True, True, True, True]])
将布尔方阵传递给Numpy的.triu函数,该函数将返回一个二维布尔蒙版,其中包含矩阵下三角的False值。
np.triu(np.ones_like(np.ones((4, 4)), dtype=bool))
array([[ True, True, True, True],
[False, True, True, True],
[False, False, True, True],
[False, False, False, True]])
然后,我们可以将其传递给Seaborn的heatmap函数,以根据此蒙版对矩阵进行可视化
ns.heatmap(matrix, mask=mask, center=0, annot=True,
fmt='.2f', square=True, cmap=cmap)
2. value_counts考虑缺失值
使用value_counts时,可将dropna设置为False来查看任何列中缺失值的比例。通过确定缺失值的比例,可以决定是否丢弃含有缺失值的记录。
#字段CouncilArea的数据分布情况
df.CouncilArea.value_counts(dropna=False, normalize=True).head()
Boroondara City Council 0.105431
Darebin City Council 0.081791
Moreland City Council 0.060877
Glen Eira City Council 0.057549
Melbourne City Council 0.056000
Name: CouncilArea, dtype: float64
但是,如果要查看所有列中缺失值的比例,则value_counts不是最佳选择。 相反,您可以执行以下操作:
-
首先,通过将缺失值的数量除以DataFrame的长度来找到比例。
-
然后,您可以过滤掉0%的列,即i。 e。 只选择缺少值的列。
missing_props = df.isna().sum() / len(df)
missing_props[missing_props > 0].sort_values(ascending=False)
BuildingArea 0.605761
YearBuilt 0.553863
Landsize 0.338813
Car 0.250394
Bathroom 0.235993
Bedroom2 0.235735
Longtitude 0.228821
Lattitude 0.228821
Price 0.218321
Propertycount 0.000086
Regionname 0.000086
CouncilArea 0.000086
Postcode 0.000029
Distance 0.000029
dtype: float64
3. 使用Pandas的Styler
我们中的许多人从未意识到pandas的巨大潜力。pandas的一个被低估且经常被忽视的功能是其对DataFrames进行样式设置的能力。 使用pandas DataFrames的.style属性,可以将条件设计和样式应用于它们。
作为第一个示例,让我们看看如何根据每个单元格的值来更改背景颜色:
diamonds = pd.read_csv('data/diamonds.csv')
diamonds.head()
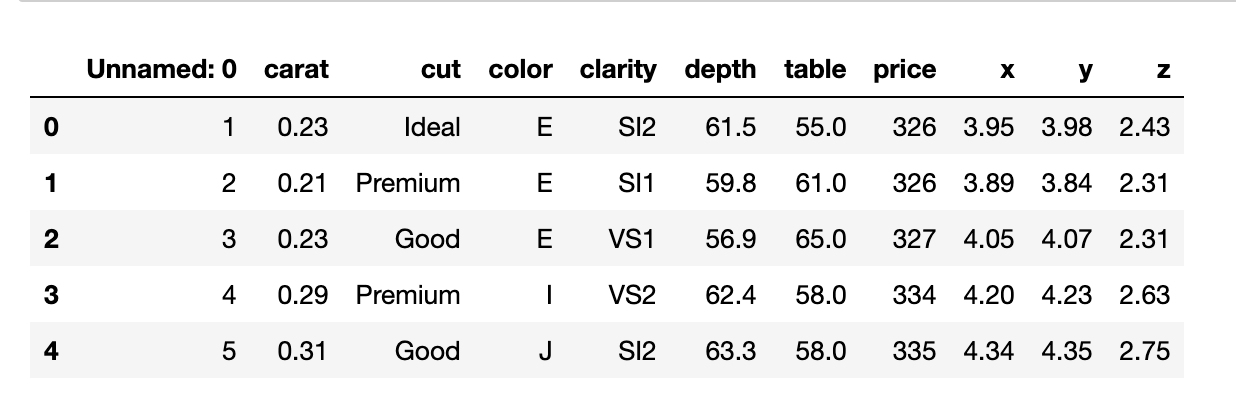
pd.crosstab(diamonds.cut, diamonds.clarity).style.background_gradient(cmap='rocket_r')
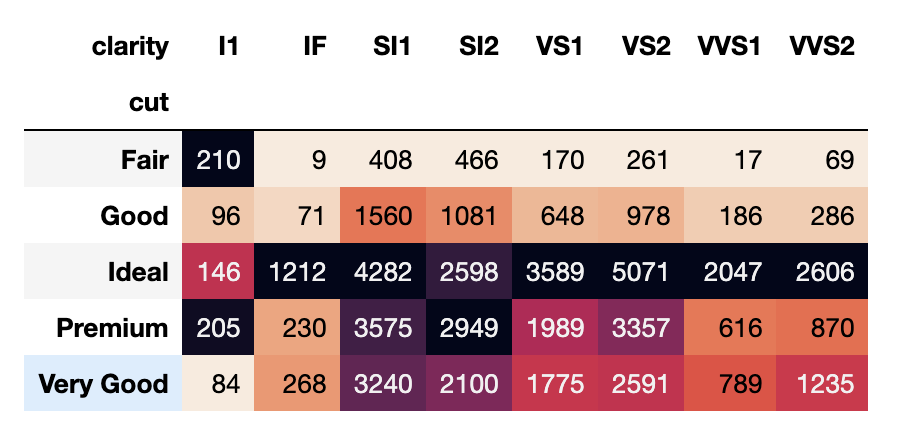
几乎没有使用Seaborn的热图功能的热图。 在这里,我们使用pd.crosstab对钻石切割(cut)和净度(clarity)的每种组合进行计数。
将.style.background_gradient与调色板配合使用,您可以轻松地发现哪种组合出现得最多。 仅从上面的DataFrame中,我们可以看到大多数钻石都是“ VS2”净度类型。
我们甚至可以通过在交叉表中找到每种钻石切割cut和净度clarity组合的平均价格来进一步做到这一点:
pd.crosstab(diamonds.cut, diamonds.clarity, aggfunc=np.mean, values=diamonds.price).style.background_gradient(cmap='rocket_r')
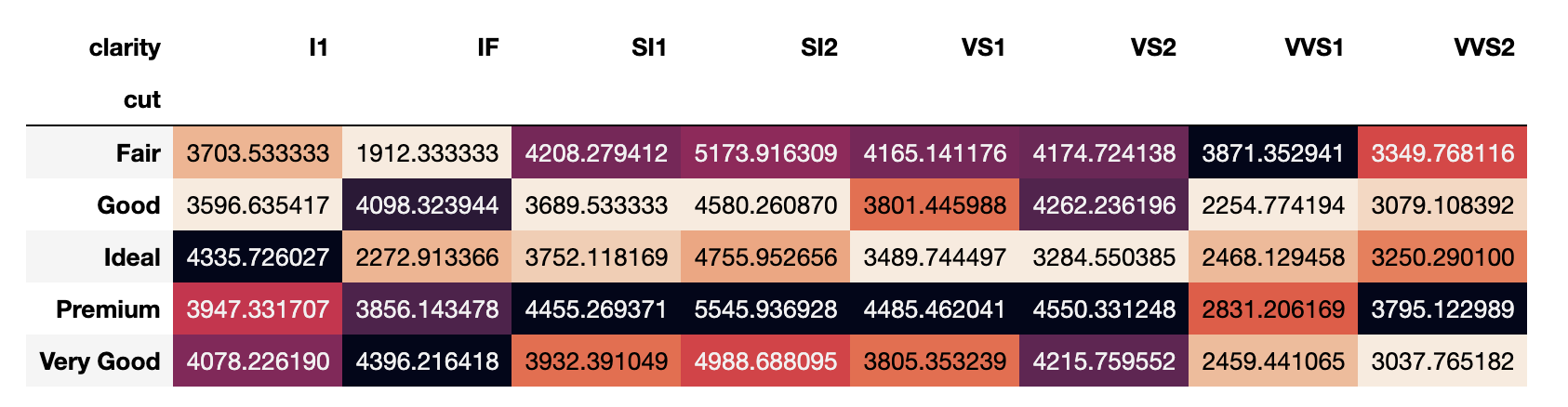
通过将.format方法与格式字符串{:.2f}链接起来,我们指定了2个浮点数的精度。
agg_prices = pd.crosstab(diamonds.cut, diamonds.clarity, aggfunc=np.mean, values=diamonds.price).style.background_gradient(cmap='rocket_r')
agg_prices.format('{:.2f}')
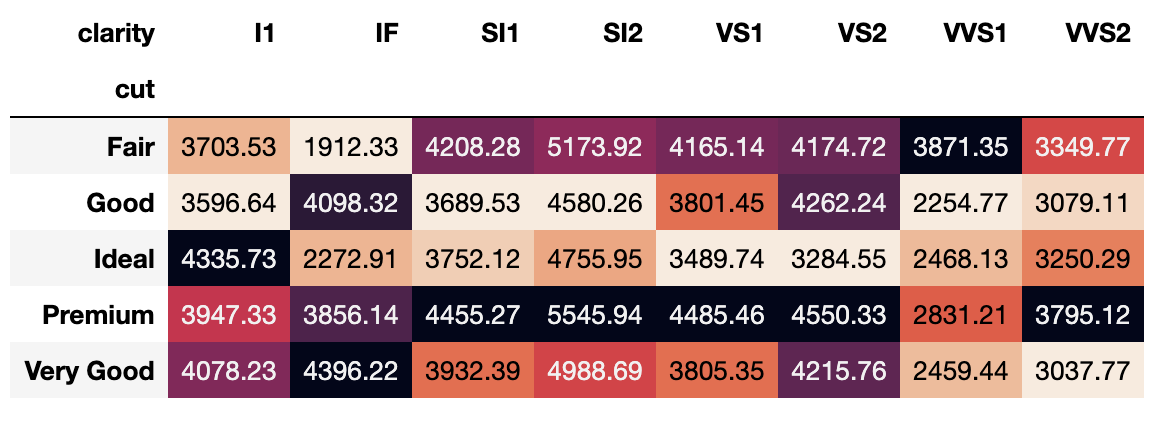
4. matplotlib默认全局设置
在进行探索性数据分析时,您可能想对所有绘图应用自定义调色板,对刻度标签使用更大的字体,更改图例的位置,使用固定的图形大小等。
对绘图自定义参数的更改是一项非常无聊,重复且耗时的任务。 幸运的是,您可以使用Matplotlib的rcParams为绘图设置全局配置。
rcParams只是一个普通的Python字典,其中包含Matplotlib的默认设置:
from matplotlib import rcParams
import warnings
warnings.filterwarnings('ignore')
rcParams
RcParams({'_internal.classic_mode': False,
'agg.path.chunksize': 0,
'animation.avconv_args': [],
'animation.avconv_path': 'avconv',
'animation.bitrate': -1,
'animation.codec': 'h264',
'animation.convert_args': [],
'animation.convert_path': 'convert',
'animation.embed_limit': 20.0,
'animation.ffmpeg_args': [],
'animation.ffmpeg_path': 'ffmpeg',
'animation.frame_format': 'png',
'animation.html': 'none',
'animation.html_args': [],
'animation.writer': 'ffmpeg',
'axes.autolimit_mode': 'data',
'axes.axisbelow': 'line',
'axes.edgecolor': 'black',
'axes.facecolor': 'white',
'axes.formatter.limits': [-7, 7],
'axes.formatter.min_exponent': 0,
'axes.formatter.offset_threshold': 4,
'axes.formatter.use_locale': False,
'axes.formatter.use_mathtext': False,
'axes.formatter.useoffset': True,
'axes.grid': False,
'axes.grid.axis': 'both',
'axes.grid.which': 'major',
'axes.labelcolor': 'black',
'axes.labelpad': 4.0,
'axes.labelsize': 'medium',
'axes.labelweight': 'normal',
'axes.linewidth': 0.8,
'axes.prop_cycle': cycler('color', ['#1f77b4', '#ff7f0e', '#2ca02c', '#d62728', '#9467bd', '#8c564b', '#e377c2', '#7f7f7f', '#bcbd22', '#17becf']),
'axes.spines.bottom': True,
'axes.spines.left': True,
'axes.spines.right': True,
'axes.spines.top': True,
'axes.titlepad': 6.0,
'axes.titlesize': 'large',
'axes.titleweight': 'normal',
'axes.unicode_minus': True,
'axes.xmargin': 0.05,
'axes.ymargin': 0.05,
'axes3d.grid': True,
'backend': 'module://ipykernel.pylab.backend_inline',
'backend_fallback': True,
'boxplot.bootstrap': None,
'boxplot.boxprops.color': 'black',
'boxplot.boxprops.linestyle': '-',
'boxplot.boxprops.linewidth': 1.0,
'boxplot.capprops.color': 'black',
'boxplot.capprops.linestyle': '-',
'boxplot.capprops.linewidth': 1.0,
'boxplot.flierprops.color': 'black',
'boxplot.flierprops.linestyle': 'none',
'boxplot.flierprops.linewidth': 1.0,
'boxplot.flierprops.marker': 'o',
'boxplot.flierprops.markeredgecolor': 'black',
'boxplot.flierprops.markeredgewidth': 1.0,
'boxplot.flierprops.markerfacecolor': 'none',
'boxplot.flierprops.markersize': 6.0,
'boxplot.meanline': False,
'boxplot.meanprops.color': 'C2',
'boxplot.meanprops.linestyle': '--',
'boxplot.meanprops.linewidth': 1.0,
'boxplot.meanprops.marker': '^',
'boxplot.meanprops.markeredgecolor': 'C2',
'boxplot.meanprops.markerfacecolor': 'C2',
'boxplot.meanprops.markersize': 6.0,
'boxplot.medianprops.color': 'C1',
'boxplot.medianprops.linestyle': '-',
'boxplot.medianprops.linewidth': 1.0,
'boxplot.notch': False,
'boxplot.patchartist': False,
'boxplot.showbox': True,
'boxplot.showcaps': True,
'boxplot.showfliers': True,
'boxplot.showmeans': False,
'boxplot.vertical': True,
'boxplot.whiskerprops.color': 'black',
'boxplot.whiskerprops.linestyle': '-',
'boxplot.whiskerprops.linewidth': 1.0,
'boxplot.whiskers': 1.5,
'contour.corner_mask': True,
'contour.negative_linestyle': 'dashed',
'datapath': '/Library/Frameworks/Python.framework/Versions/3.7/lib/python3.7/site-packages/matplotlib/mpl-data',
'date.autoformatter.day': '%Y-%m-%d',
'date.autoformatter.hour': '%m-%d %H',
'date.autoformatter.microsecond': '%M:%S.%f',
'date.autoformatter.minute': '%d %H:%M',
'date.autoformatter.month': '%Y-%m',
'date.autoformatter.second': '%H:%M:%S',
'date.autoformatter.year': '%Y',
'docstring.hardcopy': False,
'errorbar.capsize': 0.0,
'examples.directory': '',
'figure.autolayout': False,
'figure.constrained_layout.h_pad': 0.04167,
'figure.constrained_layout.hspace': 0.02,
'figure.constrained_layout.use': False,
'figure.constrained_layout.w_pad': 0.04167,
'figure.constrained_layout.wspace': 0.02,
'figure.dpi': 72.0,
'figure.edgecolor': (1, 1, 1, 0),
'figure.facecolor': (1, 1, 1, 0),
'figure.figsize': [6.0, 4.0],
'figure.frameon': True,
'figure.max_open_warning': 20,
'figure.subplot.bottom': 0.125,
'figure.subplot.hspace': 0.2,
'figure.subplot.left': 0.125,
'figure.subplot.right': 0.9,
'figure.subplot.top': 0.88,
'figure.subplot.wspace': 0.2,
'figure.titlesize': 'large',
'figure.titleweight': 'normal',
'font.cursive': ['Apple Chancery',
'Textile',
'Zapf Chancery',
'Sand',
'Script MT',
'Felipa',
'cursive'],
'font.family': ['sans-serif'],
'font.fantasy': ['Comic Sans MS',
'Chicago',
'Charcoal',
'Impact',
'Western',
'Humor Sans',
'xkcd',
'fantasy'],
'font.monospace': ['DejaVu Sans Mono',
'Bitstream Vera Sans Mono',
'Computer Modern Typewriter',
'Andale Mono',
'Nimbus Mono L',
'Courier New',
'Courier',
'Fixed',
'Terminal',
'monospace'],
'font.sans-serif': ['DejaVu Sans',
'Bitstream Vera Sans',
'Computer Modern Sans Serif',
'Lucida Grande',
'Verdana',
'Geneva',
'Lucid',
'Arial',
'Helvetica',
'Avant Garde',
'sans-serif'],
'font.serif': ['DejaVu Serif',
'Bitstream Vera Serif',
'Computer Modern Roman',
'New Century Schoolbook',
'Century Schoolbook L',
'Utopia',
'ITC Bookman',
'Bookman',
'Nimbus Roman No9 L',
'Times New Roman',
'Times',
'Palatino',
'Charter',
'serif'],
'font.size': 10.0,
'font.stretch': 'normal',
'font.style': 'normal',
'font.variant': 'normal',
'font.weight': 'normal',
'grid.alpha': 1.0,
'grid.color': '#b0b0b0',
'grid.linestyle': '-',
'grid.linewidth': 0.8,
'hatch.color': 'black',
'hatch.linewidth': 1.0,
'hist.bins': 10,
'image.aspect': 'equal',
'image.cmap': 'viridis',
'image.composite_image': True,
'image.interpolation': 'nearest',
'image.lut': 256,
'image.origin': 'upper',
'image.resample': True,
'interactive': True,
'keymap.all_axes': ['a'],
'keymap.back': ['left', 'c', 'backspace', 'MouseButton.BACK'],
'keymap.copy': ['ctrl+c', 'cmd+c'],
'keymap.forward': ['right', 'v', 'MouseButton.FORWARD'],
'keymap.fullscreen': ['f', 'ctrl+f'],
'keymap.grid': ['g'],
'keymap.grid_minor': ['G'],
'keymap.help': ['f1'],
'keymap.home': ['h', 'r', 'home'],
'keymap.pan': ['p'],
'keymap.quit': ['ctrl+w', 'cmd+w', 'q'],
'keymap.quit_all': ['W', 'cmd+W', 'Q'],
'keymap.save': ['s', 'ctrl+s'],
'keymap.xscale': ['k', 'L'],
'keymap.yscale': ['l'],
'keymap.zoom': ['o'],
'legend.borderaxespad': 0.5,
'legend.borderpad': 0.4,
'legend.columnspacing': 2.0,
'legend.edgecolor': '0.8',
'legend.facecolor': 'inherit',
'legend.fancybox': True,
'legend.fontsize': 'medium',
'legend.framealpha': 0.8,
'legend.frameon': True,
'legend.handleheight': 0.7,
'legend.handlelength': 2.0,
'legend.handletextpad': 0.8,
'legend.labelspacing': 0.5,
'legend.loc': 'best',
'legend.markerscale': 1.0,
'legend.numpoints': 1,
'legend.scatterpoints': 1,
'legend.shadow': False,
'legend.title_fontsize': None,
'lines.antialiased': True,
'lines.color': 'C0',
'lines.dash_capstyle': 'butt',
'lines.dash_joinstyle': 'round',
'lines.dashdot_pattern': [6.4, 1.6, 1.0, 1.6],
'lines.dashed_pattern': [3.7, 1.6],
'lines.dotted_pattern': [1.0, 1.65],
'lines.linestyle': '-',
'lines.linewidth': 1.5,
'lines.marker': 'None',
'lines.markeredgecolor': 'auto',
'lines.markeredgewidth': 1.0,
'lines.markerfacecolor': 'auto',
'lines.markersize': 6.0,
'lines.scale_dashes': True,
'lines.solid_capstyle': 'projecting',
'lines.solid_joinstyle': 'round',
'markers.fillstyle': 'full',
'mathtext.bf': 'sans:bold',
'mathtext.cal': 'cursive',
'mathtext.default': 'it',
'mathtext.fallback_to_cm': True,
'mathtext.fontset': 'dejavusans',
'mathtext.it': 'sans:italic',
'mathtext.rm': 'sans',
'mathtext.sf': 'sans',
'mathtext.tt': 'monospace',
'patch.antialiased': True,
'patch.edgecolor': 'black',
'patch.facecolor': 'C0',
'patch.force_edgecolor': False,
'patch.linewidth': 1.0,
'path.effects': [],
'path.simplify': True,
'path.simplify_threshold': 0.1111111111111111,
'path.sketch': None,
'path.snap': True,
'pdf.compression': 6,
'pdf.fonttype': 3,
'pdf.inheritcolor': False,
'pdf.use14corefonts': False,
'pgf.preamble': '',
'pgf.rcfonts': True,
'pgf.texsystem': 'xelatex',
'polaraxes.grid': True,
'ps.distiller.res': 6000,
'ps.fonttype': 3,
'ps.papersize': 'letter',
'ps.useafm': False,
'ps.usedistiller': False,
'savefig.bbox': None,
'savefig.directory': '~',
'savefig.dpi': 'figure',
'savefig.edgecolor': 'white',
'savefig.facecolor': 'white',
'savefig.format': 'png',
'savefig.frameon': True,
'savefig.jpeg_quality': 95,
'savefig.orientation': 'portrait',
'savefig.pad_inches': 0.1,
'savefig.transparent': False,
'scatter.edgecolors': 'face',
'scatter.marker': 'o',
'svg.fonttype': 'path',
'svg.hashsalt': None,
'svg.image_inline': True,
'text.antialiased': True,
'text.color': 'black',
'text.hinting': 'auto',
'text.hinting_factor': 8,
'text.latex.preamble': '',
'text.latex.preview': False,
'text.latex.unicode': True,
'text.usetex': False,
'timezone': 'UTC',
'tk.window_focus': False,
'toolbar': 'toolbar2',
'verbose.fileo': 'sys.stdout',
'verbose.level': 'silent',
'webagg.address': '127.0.0.1',
'webagg.open_in_browser': True,
'webagg.port': 8988,
'webagg.port_retries': 50,
'xtick.alignment': 'center',
'xtick.bottom': True,
'xtick.color': 'black',
'xtick.direction': 'out',
'xtick.labelbottom': True,
'xtick.labelsize': 'medium',
'xtick.labeltop': False,
'xtick.major.bottom': True,
'xtick.major.pad': 3.5,
'xtick.major.size': 3.5,
'xtick.major.top': True,
'xtick.major.width': 0.8,
'xtick.minor.bottom': True,
'xtick.minor.pad': 3.4,
'xtick.minor.size': 2.0,
'xtick.minor.top': True,
'xtick.minor.visible': False,
'xtick.minor.width': 0.6,
'xtick.top': False,
'ytick.alignment': 'center_baseline',
'ytick.color': 'black',
'ytick.direction': 'out',
'ytick.labelleft': True,
'ytick.labelright': False,
'ytick.labelsize': 'medium',
'ytick.left': True,
'ytick.major.left': True,
'ytick.major.pad': 3.5,
'ytick.major.right': True,
'ytick.major.size': 3.5,
'ytick.major.width': 0.8,
'ytick.minor.left': True,
'ytick.minor.pad': 3.4,
'ytick.minor.right': True,
'ytick.minor.size': 2.0,
'ytick.minor.visible': False,
'ytick.minor.width': 0.6,
'ytick.right': False})
您可以调整每个图的任意参数设置,一般的图像设置如固定图形大小,刻度标签字体大小以及其他一些参数。
通过这种设置,可以减少很多重复的代码量
# 去掉顶部和右侧的线条Remove top and right spines
rcParams['axes.spines.top'] = False
rcParams['axes.spines.right'] = False
# 设置图的尺寸Set fixed figure size
rcParams['figure.figsize'] = [12, 9]
# 设置图片像素清晰度 Set dots per inch to 300, very high quality images
rcParams['figure.dpi'] = 300
# 设置自动调整布局Enable autolayout
rcParams['figure.autolayout'] = True
# 设置全局字号Set global fontsize
rcParams['font.style'] = 16
# 刻度字号Fontsize of ticklabels
rcParams['xtick.labelsize'] = 10
rcParams['ytick.labelsize'] = 10
5. Pandas全局设置
就像Matplotlib一样,pandas具有可以使用的全局设置。 当然,它们大多数与显示选项有关。
- get_option() - 获取pandas单个选项
- set_option() — 设置pandas单个选项
- reset_option() — 重置pandas选项值
我更喜欢显示所有的列,lets go
pd.set_option('display.max_columns', None)
df.head()
